The field of technology and AI has witnessed unparalleled growth due to the introduction of a multitude of applications fueled by deep learning. As a subfield of machine learning, deep learning proves adept at solving intricate problems in the technical realm.
In this blog, VNG Cloud will explore the diverse facets of deep learning, delving into its advantages, disadvantages, and its wide-ranging applications. Understanding the nuanced interaction among these components is pivotal for unlocking the complete potential of deep learning in our increasingly technology-driven world.
Advantages of Deep Learning
Deep learning offers numerous advantages compared to traditional machine learning methods. Here are the top 10 advantages of deep learning:
Automatic feature learning
Deep learning algorithms possess the ability to automatically learn features from data, eliminating the need for manual feature engineering. This proves especially valuable in tasks where defining features is challenging, such as in image recognition.
Handling large and complex data
Deep learning algorithms excel in processing large and intricate datasets, a task often challenging for traditional machine learning algorithms. This capability is instrumental in extracting valuable insights from extensive datasets, making deep learning a powerful tool for big data analytics.
Improved performance
Demonstrating high performance across a spectrum of problems, including image and speech recognition, natural language processing, and computer vision, deep learning algorithms showcase superior performance compared to traditional methods.
Handling non-linear relationships
Deep learning unravels non-linear relationships within data that may be elusive to detect using conventional methods, contributing to a more accurate representation of complex patterns.
Handling structured and unstructured data
Deep learning algorithms exhibit versatility by effectively handling both structured and unstructured data types, such as images, text, and audio, expanding their applicability across diverse domains.
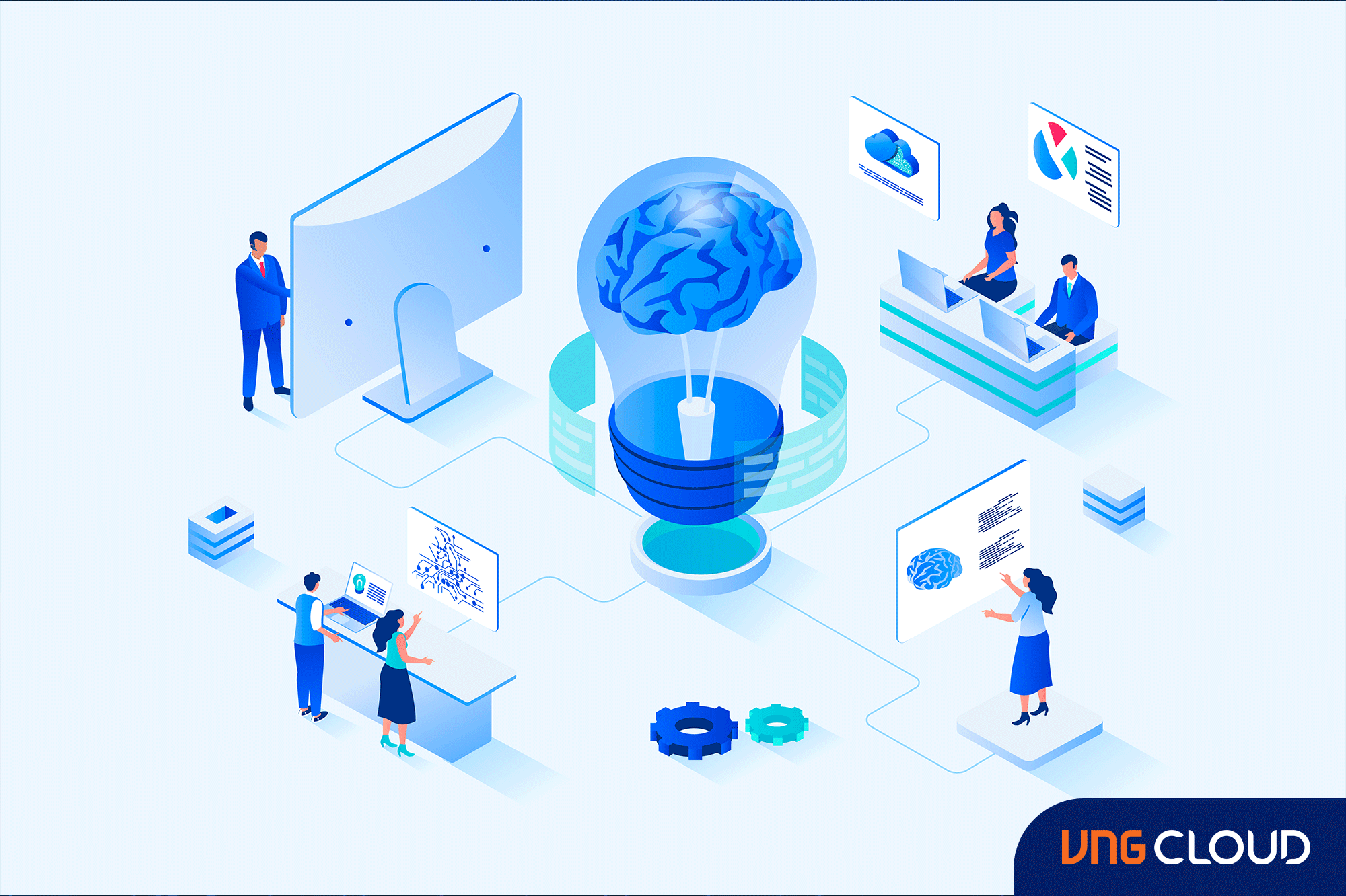
Predictive modeling
Deep learning serves as a potent tool for predictive modeling, enabling organizations to forecast future events or trends. This capability aids in strategic decision-making and future planning.
Handling missing data
Deep learning algorithms display resilience in the face of missing data, still providing meaningful predictions. This is particularly advantageous in real-world scenarios where data is often incomplete.
Handling sequential data
Specialized deep learning algorithms like Recurrent Neural Networks (RNNs) and Long Short-term Memory (LSTM) networks are adept at handling sequential data, such as time series, speech, and text. Their capacity to maintain context and memory over time allows for informed predictions based on historical inputs.
Scalability
Deep learning models are easily scalable, accommodating increasing volumes of data. They can be efficiently deployed on cloud platforms and edge devices, offering flexibility and accessibility.
Generalization
Deep learning models demonstrate a strong ability to generalize to new situations or contexts. Their capacity to learn abstract and hierarchical representations of data contributes to their adaptability in diverse scenarios.
Deep learning's advantages, ranging from automatic feature learning to generalization ability, makes it a game-changer in the world of machine learning.
Disadvantages of Deep Learning
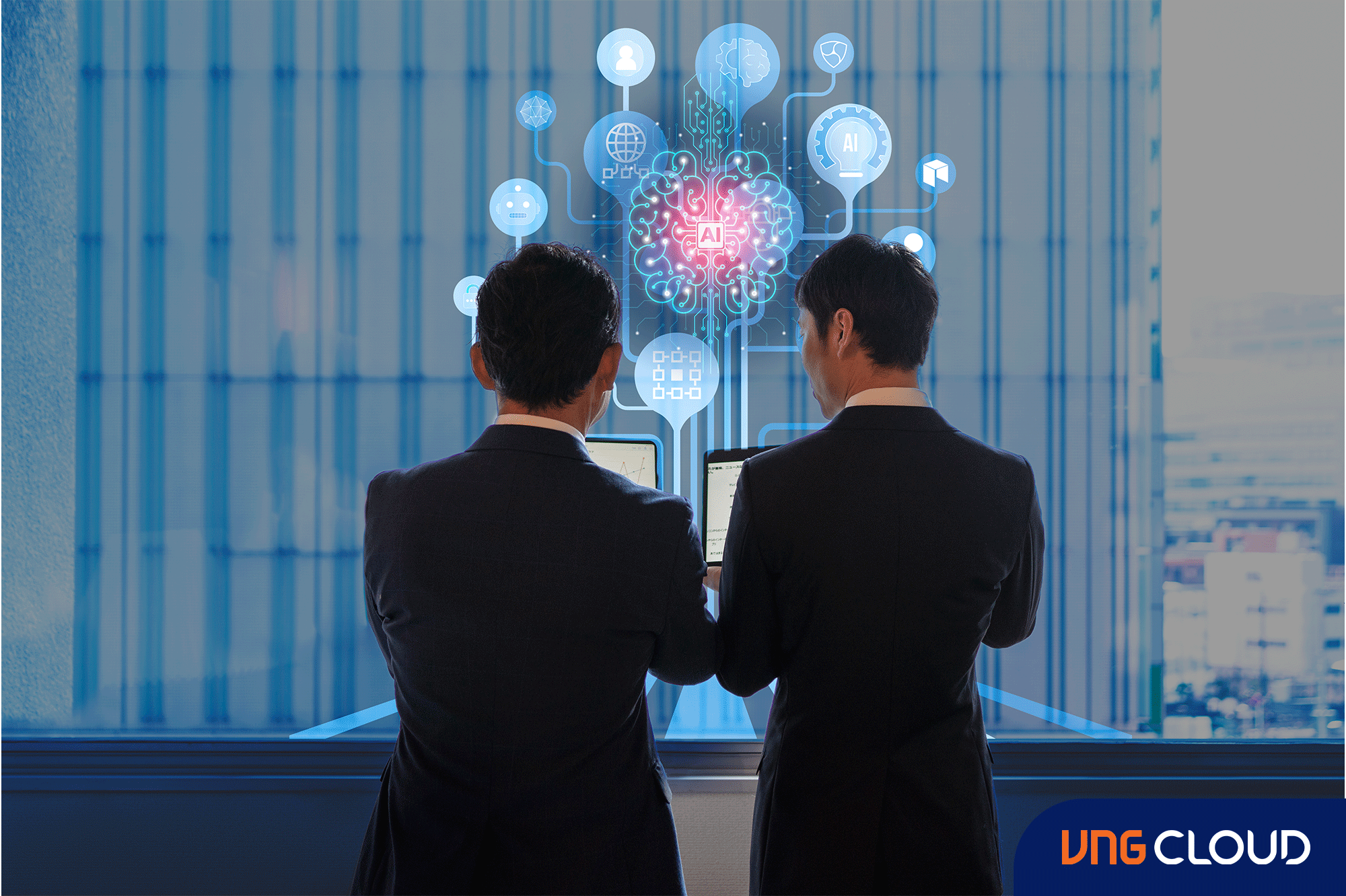
Although deep learning offers several advantages, it also requires consideration of the top 9 drawbacks:
High computational cost
Training deep learning models demands substantial computational resources, involving powerful GPUs and extensive memory, which can be expensive and time-consuming.
Overfitting
Overfitting occurs when a model becomes too specialized in the training data, leading to poor performance on new, unseen data. This is a common issue in deep learning, particularly with large neural networks, and can result from factors like insufficient data, model complexity, or a lack of normalization.
Lack of interpretability
Deep learning models, especially those with many layers, can be intricate and challenging to interpret. Understanding how the model makes predictions and identifying errors or biases can be difficult.
Dependence on data quality
The performance of deep learning algorithms relies heavily on the quality of the training data. Noisy, incomplete, or biased data can adversely affect the model's accuracy.
Data privacy and security concerns
Deep learning models often rely on large datasets, raising concerns about data privacy and security. Misuse of data by malicious actors can lead to serious consequences, such as identity theft and invasion of privacy.
Lack of domain expertise
Successful implementation of deep learning requires a thorough understanding of the domain and the specific problem being addressed. Insufficient domain expertise can hinder problem formulation and algorithm selection.
Unforeseen consequences
Deep learning models may lead to unintended consequences, such as biased predictions that discriminate against certain groups, raising ethical concerns.
Limited to the data on which it was trained
Deep learning models can only make predictions based on the data they were trained on, limiting their ability to generalize to new situations or contexts not represented in the training data.
Black box models
Some deep learning models are considered "black box", as it is challenging to understand how the model makes predictions and identify the factors influencing those predictions.
While deep learning has significant advantages, it is crucial to be mindful of these limitations when applying it to problem-solving scenarios. Understanding both the strengths and weaknesses is essential for making informed decisions and ensuring responsible and effective use of deep learning techniques.
Application of Deep Learning
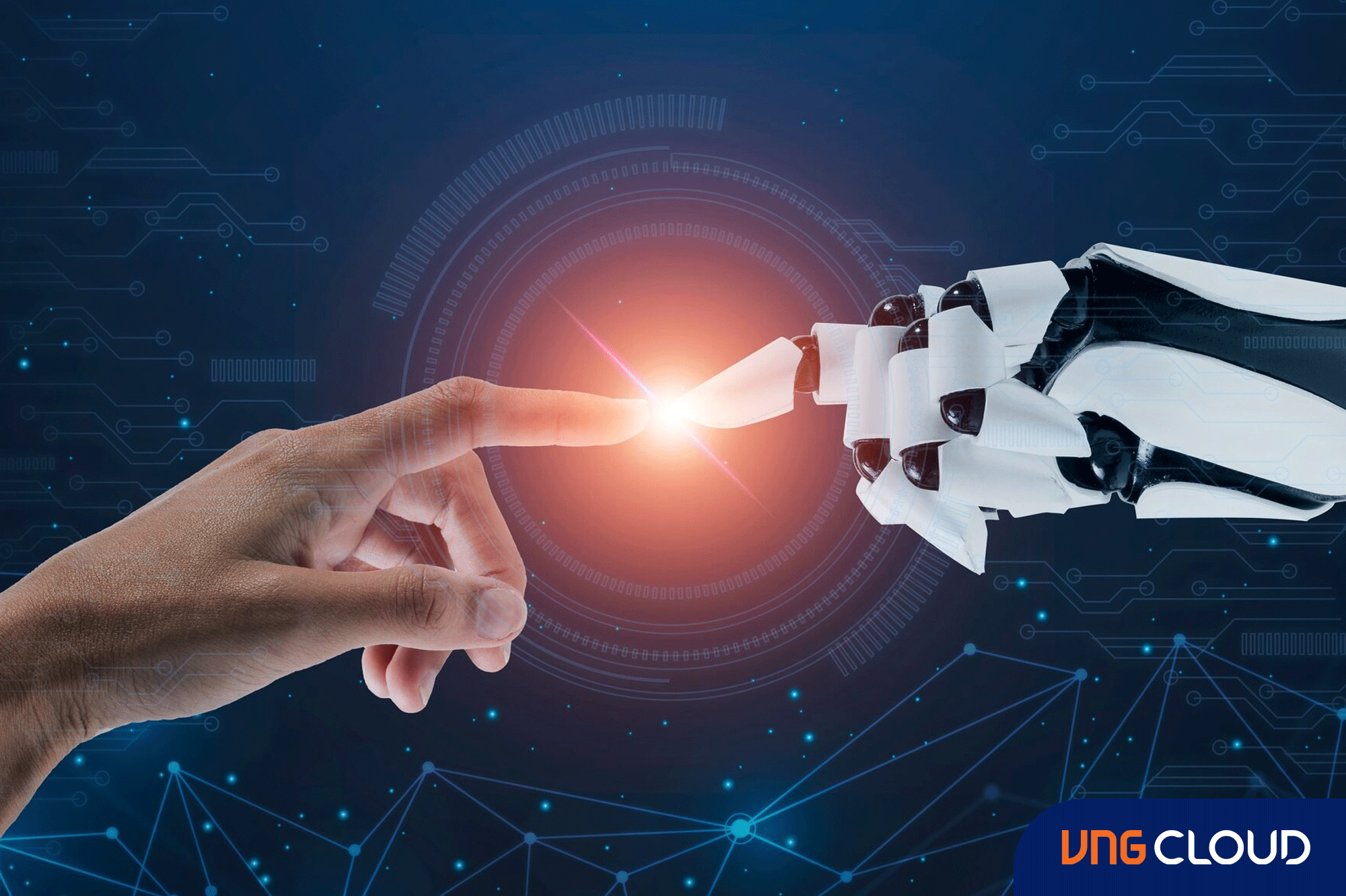
Deep learning finds a myriad of applications across diverse industries and fields. Some of the most common applications include:
Computer vision
Deep learning is employed in tasks such as image and video recognition, object detection, and semantic segmentation. Applications span self-driving cars, security cameras, and image recognition for mobile devices.
Natural language processing (NLP)
In natural language understanding, machine translation, and sentiment analysis, deep learning plays a crucial role. Applications encompass chatbots, virtual assistants, and language-based search engines.
Speech recognition
Deep learning is utilized in speech recognition, voice identification, and voice synthesis, powering applications like voice-controlled assistants, voice-enabled devices, and voice-controlled robots.
Predictive analytics
Analyzing historical data for predicting future events is a key application of deep learning. This is seen in fraud detection, customer churn prediction, and demand forecasting.
Healthcare
Deep learning is instrumental in analyzing medical images and patient data for improved diagnosis and treatment. Applications include cancer diagnosis, drug discovery, and personalized medicine.
Finance
In finance, deep learning is used for detecting fraudulent transactions, identifying potential risks, and making predictions about stock prices. This involves applications like credit fraud detection, algorithmic trading, and risk management.
Marketing
Analyzing customer data, predicting customer behavior, and personalizing marketing campaigns are areas where deep learning is applied. Applications include customer segmentation, customer lifetime value prediction, and personalization.
Gaming
Deep learning is employed to train agents in playing games and developing intelligent game-playing algorithms. Applications include game bots, game-playing AI, and adaptive game design.
Robotics
Enabling robots to learn from experience and adapt to their environment is facilitated by deep learning. Applications include autonomous vehicles, drones, and industrial robots.
Cybersecurity
Deep learning plays a crucial role in detecting patterns in network traffic and responding to cyber threats. Applications include intrusion detection and prevention, as well as malware detection.
These examples represent just a fraction of the diverse applications of deep learning today. The field is continually evolving, with new applications emerging as technology and data availability improve.
Final thoughts
The substantial contribution of deep learning is evident in the proliferation of AI-powered applications and technologies. Today, deep learning serves as the foundation for numerous applications. It is crucial to highlight the disadvantages of deep learning to enable a more informed and strategic utilization of this powerful technology.