Deep learning, a swiftly advancing subset of machine learning, has achieved noteworthy progress in the realm of artificial intelligence (AI) by adeptly capturing intricate data relationships. This technique involves the training of artificial neural networks comprising multiple layers, enabling them to glean insights from extensive datasets. Consequently, these networks excel in making precise predictions, classifying information, and executing intricate tasks more effectively than conventional machine learning algorithms. The widespread applications of deep learning span diverse industries, including healthcare, finance, agriculture, and natural language processing, signifying its transformative impact.
Fortunately, numerous deep learning frameworks, including PyTorch and TensorFlow, offer the flexibility for individuals to develop their own deep learning models and conduct experiments in this dynamic field.
This article delves into six prevalent applications of deep learning, namely computer vision, natural language processing, healthcare, finance, agriculture, and cybersecurity. We will discuss how deep learning is being used in these fields and the benefits it provides.
1. Computer Vision
Many artificial intelligence applications owe their existence to the strides made in computer vision, with deep learning playing a pivotal role in advancing this field. Notably, it has propelled developments in object detection, image classification, and image segmentation within computer vision.
For instance, deep learning algorithms can be trained to identify specific elements within an image, such as people or objects. This capability proves indispensable in applications like autonomous driving and robotics, where vehicles or robots need to discern and react to diverse objects in their surroundings. In healthcare, deep learning aids in the analysis of medical images, showcasing its relevance across various domains.
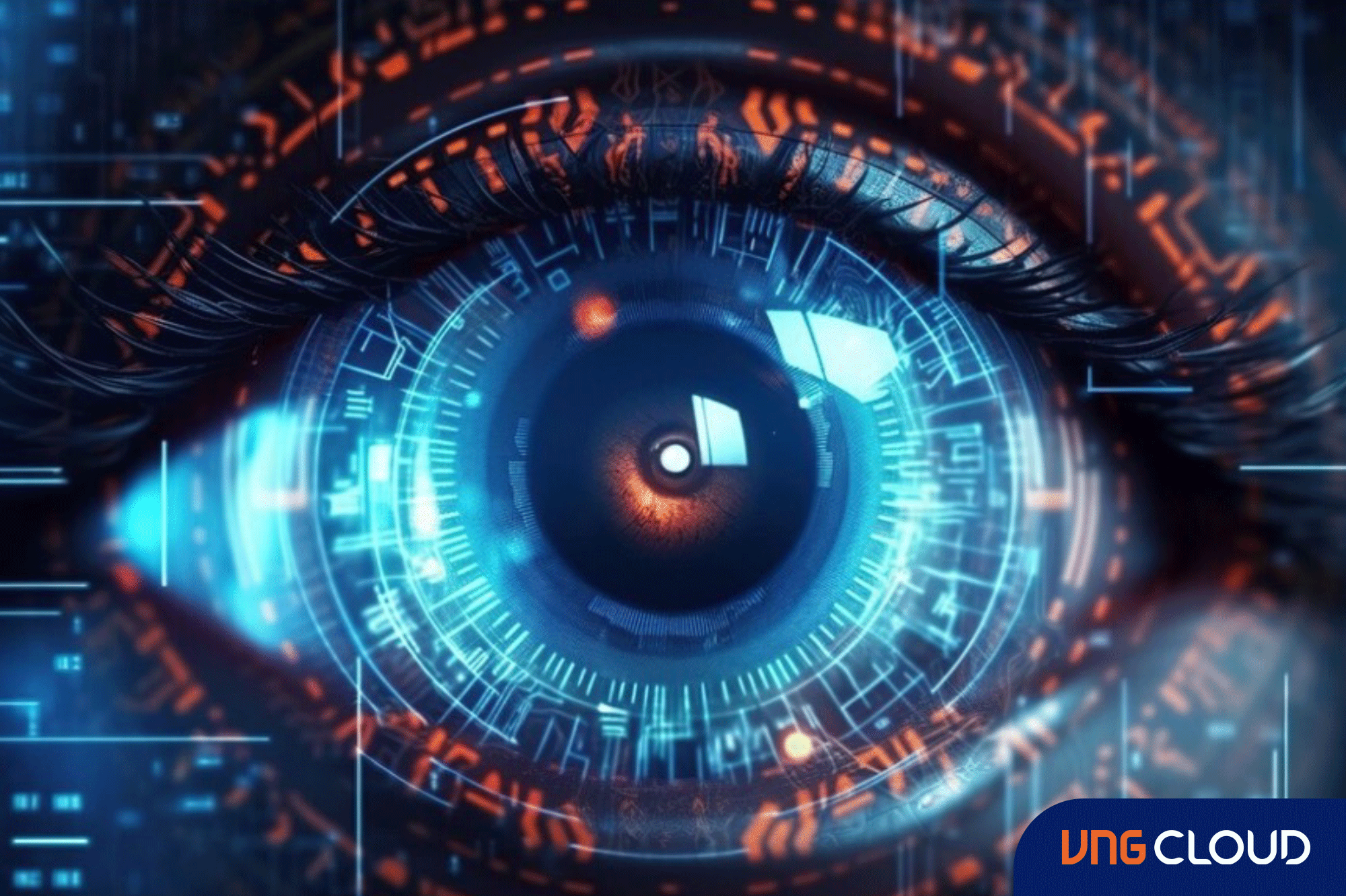
Moreover, deep learning contributes to the creation of more immersive virtual and augmented reality experiences. Through training neural networks on extensive datasets of images and videos, developers craft virtual environments that respond realistically to user inputs. This technology finds applications in diverse fields, including video games, training simulations, and architectural design, among others.
In essence, deep learning revolutionizes computer vision by empowering machines to comprehend and interpret visual information in ways that were once deemed impossible.
2. Natural Language Processing (NLP)
Natural Language Processing (NLP) is the domain that explores the interaction between human language and computers. Deep learning has revolutionized NLP, empowering computers to comprehend and respond to human language effectively. A prominent application of deep learning in NLP is machine translation, where models are trained on extensive datasets of human language to accurately translate text from one language to another. This innovation finds diverse applications, such as website localization, document translation, and language learning tools.
Another significant use of deep learning in NLP is sentiment analysis. This involves scrutinizing text to discern the emotional tone or sentiment expressed by the author. Deep learning models excel in accurately classifying text as positive, negative, or neutral. This capability is valuable for businesses seeking insights into customer opinions and feedback on their products or services, aiding them in making informed decisions and enhancing customer satisfaction.
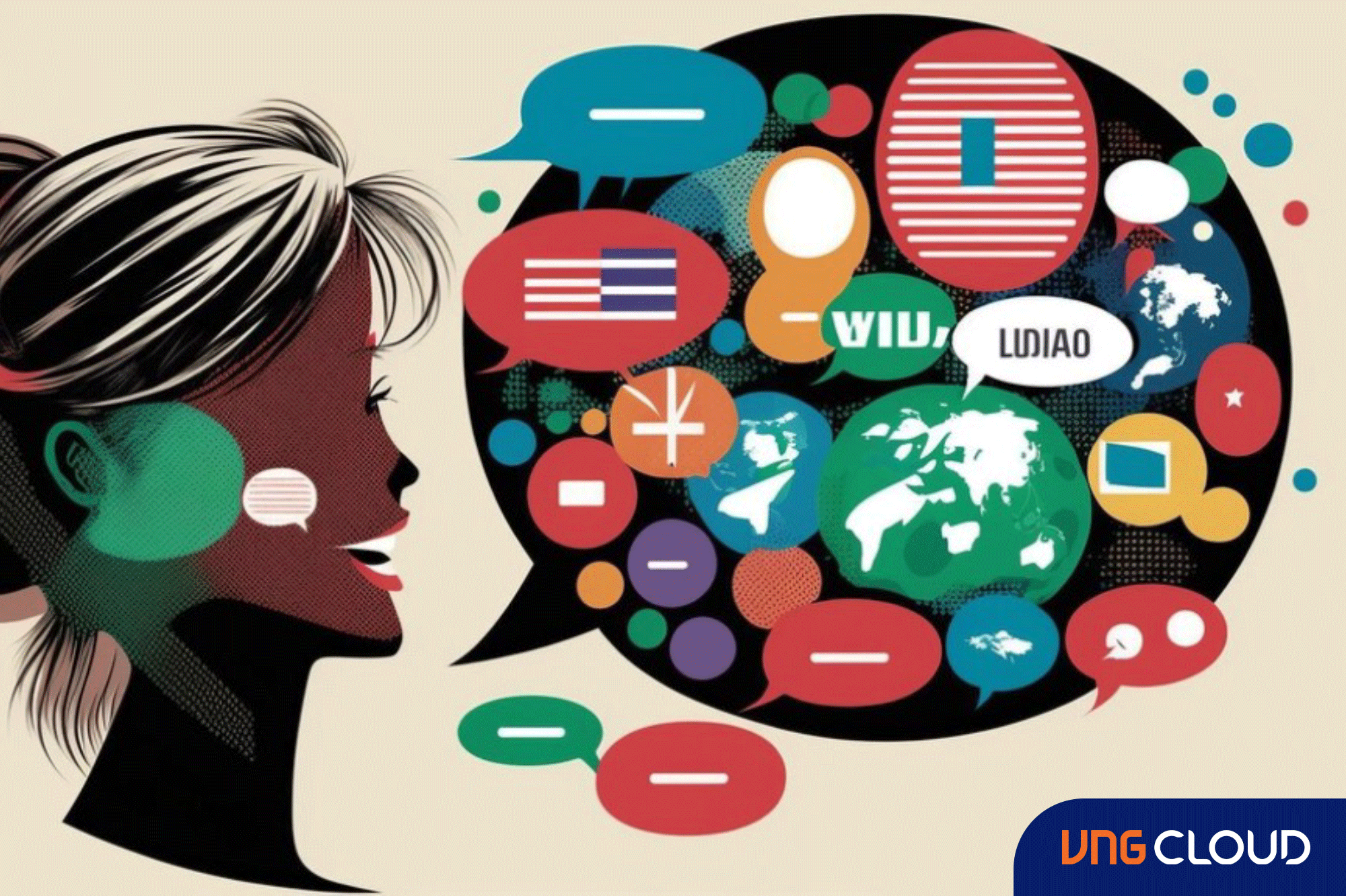
3. Application in Healthcare
Deep learning is a game-changer in healthcare, particularly in enhancing medical imaging such as CT and MRI scans, enabling specialists to formulate more precise and personalized treatment plans. For instance, deep learning algorithms can be used to analyze medical images like X-rays and MRIs, uncovering abnormalities that may elude the naked eye. Additionally, deep learning contributes to the realm of personalized medicine, tailoring treatments to an individual's unique genetic composition.
The capacity of deep learning algorithms to analyze extensive medical data enables the identification of intricate patterns and trends challenging for human physicians to discern. Examples include the early detection of cancer in mammograms, identification of blood clots in brain scans, and diagnosis of degenerative diseases in spinal cord images. Consequently, this innovation has spurred the creation of novel diagnostic tools and treatment modalities for conditions such as cancer, heart disease, and neurological disorders.
Overall, the potential of deep learning to revolutionize healthcare is vast, offering improvements in diagnostics, treatment methodologies, and ultimately, patient outcomes. As technology continues to advance, we anticipate witnessing further innovative applications of deep learning within the healthcare domain.
4. Application in Finance
Deep learning stands as a formidable force in revolutionizing the financial sector, offering a diverse array of applications that enhance decision-making, risk management, fraud detection, and customer experience. A pivotal application lies in fraud detection, where deep learning models are adeptly trained to recognize fraudulent transactions, mitigating false positives and enhancing overall accuracy. This not only safeguards financial institutions from significant losses but also fosters customer trust and satisfaction.
Risk management is another prominent domain where deep learning makes its mark in finance. By leveraging these models to scrutinize vast datasets, financial institutions can discern patterns and correlations that aid in predicting future risks and uncovering potential opportunities. This informed decision-making helps mitigate risks and enhances overall strategic planning. Furthermore, deep learning contributes to an improved customer experience by elevating the precision and efficiency of analyzing customer data, allowing for the identification of behavior patterns.
For instance, deep learning models can predict customer churn and facilitate the delivery of targeted offers and promotions, thereby retaining customers and bolstering satisfaction.
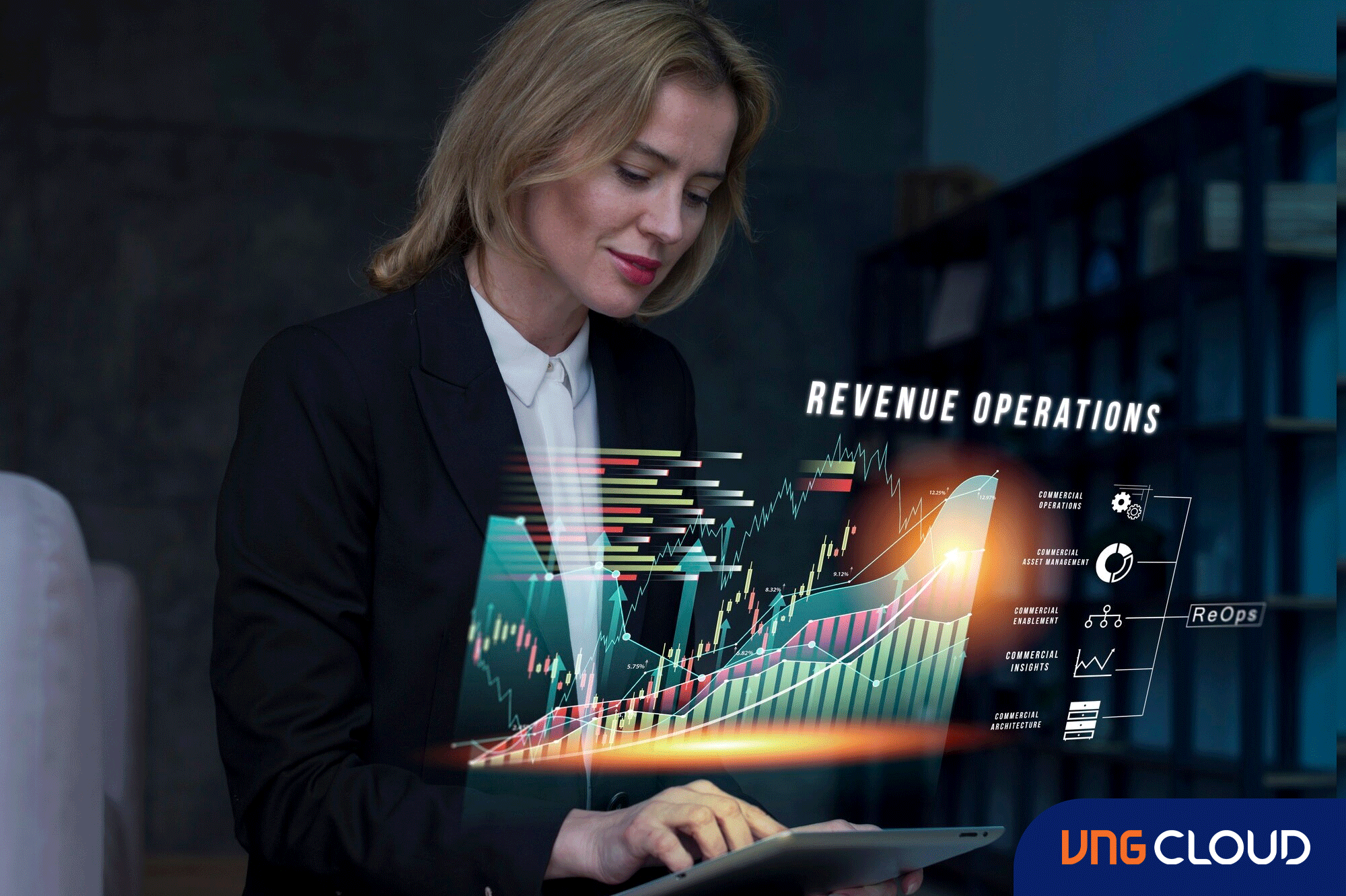
5. Application in Agriculture
Deep learning empowers farmers with the tools to make informed decisions and enhance crop yields. A pivotal application lies in crop monitoring, where deep learning models are trained to scrutinize satellite, drone, and other remote sensing data to detect changes in crop health and predict yields. This insight enables the identification of specific areas in the field requiring attention, such as those in need of irrigation or fertilization.
Precision farming represents another significant application of deep learning in agriculture. This approach leverages data analytics and technology to optimize crop yield while minimizing waste. Deep learning models analyze data on variables like soil moisture, temperature, and nutrient levels, providing recommendations on when and where to plant, irrigate, and fertilize crops. This optimization aids farmers in maximizing resources, reducing costs, and ultimately improving the overall health and yield of their crops.
6. Application in Cybersecurity
Deep learning is a valuable asset in bolstering threat detection and response within cybersecurity. The escalating sophistication of cybersecurity threats necessitates more advanced approaches, as conventional rule-based methods often fall short. Deep learning algorithms, capable of scrutinizing extensive datasets, excel in identifying patterns and anomalies that may signal a security breach or impending attack.
In the realm of cybersecurity, one significant application of deep learning is intrusion detection. Intrusion detection systems (IDS) enable the monitoring of network traffic, identifying suspicious activity indicative of a potential attack. Training deep learning algorithms on substantial datasets of network traffic facilitates the detection of patterns and anomalies associated with attacks, leading to swifter and more effective responses compared to traditional rule-based methods.
Additionally, deep learning proves instrumental in the development of malware detection systems. Malware, a malicious software type employed to compromise computer systems and pilfer data, poses a constant threat. Deep learning algorithms proficiently recognize patterns that hint at the presence of malware, enabling the detection and prevention of malware attacks before they can inflict harm.
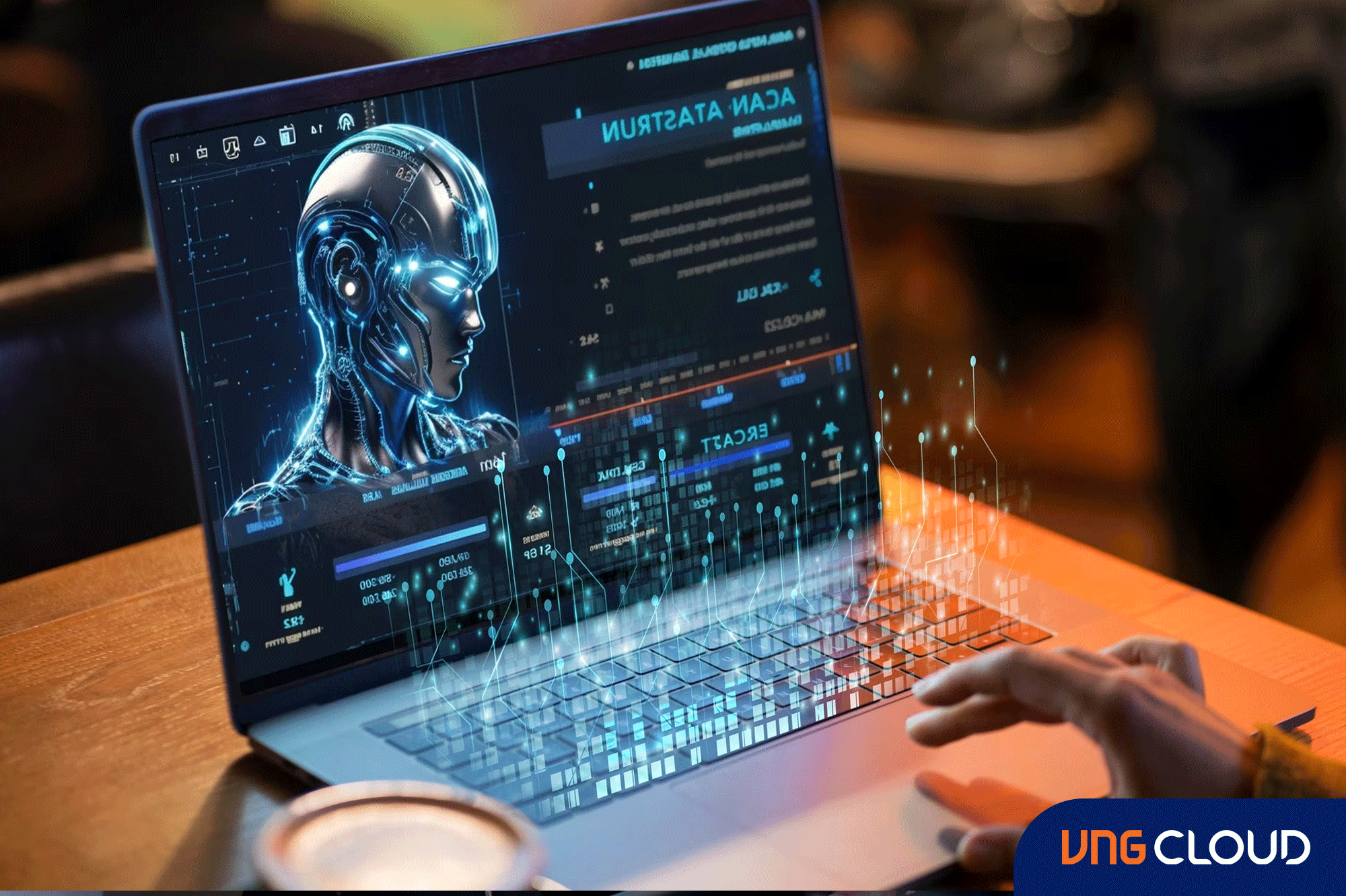
Conclusion
In conclusion, the present exploration of deep learning's applications across diverse fields underscores its transformative influence on technology. From reshaping healthcare through precise diagnostics to revolutionizing agriculture with data-driven valuable insights, and from fortifying cybersecurity against sophisticated threats to refining financial decision-making, deep learning stands as a powerful catalyst for innovation.
As we stand at the forefront of this technological evolution, it is evident that the trajectory of deep learning is bound for even more groundbreaking applications in the future. The ever-expanding capabilities of this dynamic field hold the promise of addressing complex challenges, driving efficiency, and unveiling new possibilities across industries. As deep learning continues to evolve, we can anticipate witnessing increasingly innovative and impactful applications that will redefine the way we approach and solve problems in the years to come.